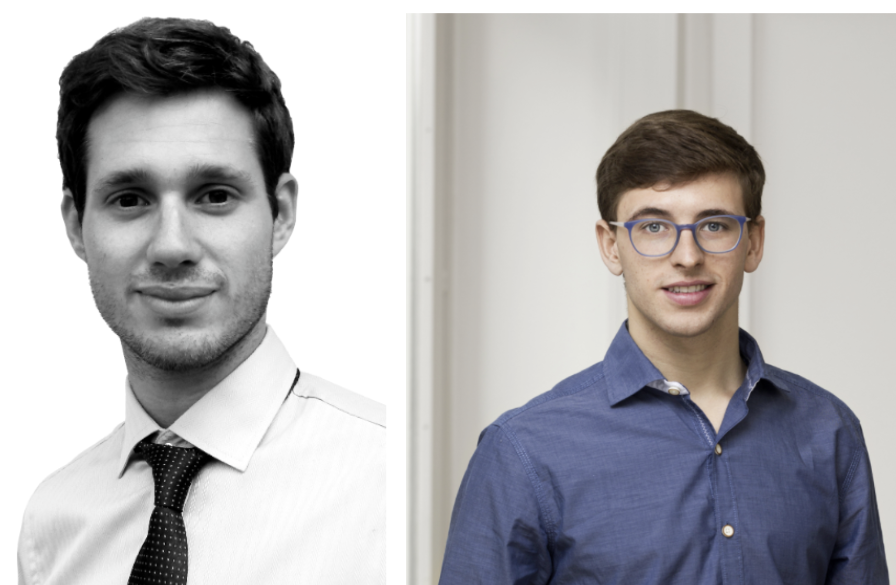
🔬 Research Summary by Nicolò Pagan and Nicolas Lanzetti.
[Original paper by Nicolas Lanzetti, Florian Dörfler, and Nicolò Pagan]
Overview: Social media are nowadays important drivers of information, yet personalization plays a huge role in determining the type of content users are served. Furthermore, how users interact with the content, e.g., read, like, or comment, effectively creates a closed loop between opinion formation and content recommendation. In this paper, we study the opinion-steering effects of this closed loop and uncover a fundamental discrepancy between the individual (microscopic) level and the aggregate/population (macroscopic) level.
Introduction
In the U.S. presidential elections of 1960 and 1964, the Democratic Party secured victory, reflecting a seemingly stable national-level aggregate preference. Yet, a closer inspection of regional voting patterns unveils a different story. Notably, most western states, including California, Oregon, and Washington, which had previously leaned Republican, shifted their allegiance to the Democrats. Conversely, some traditionally Democratic southern states, such as Alabama, Georgia, and South Carolina, transitioned to the Republican camp. These shifts reveal that macroscopic observations could mask significant microscopic patterns.
With this example in mind, we delve into the realm of content personalization, a ubiquitous practice in today’s digital landscape that is known to influence users’ opinions significantly. Our journey begins with constructing a straightforward parametric model capturing the closed loop between opinion formation and content personalization. Through our analysis, we uncover the role of trade-offs between content exploration and exploitation in shaping the differences between microscopic and macroscopic effects. In particular, it explains when and why seemingly steady collective opinion distributions, derived from aggregate and anonymized data, can mask substantial individual opinion shifts, underscoring the need for a more nuanced understanding of these phenomena.
Key Insights
An Illustrative Example: Do “Micro” and “Macro” Always Align?
Let’s start with an example. Consider a population of identical agents whereby each agent has an opinion, captured by a real number, on a given topic. An example is the user’s ideological position, where negative values represent liberal positions and positive values represent more conservative positions.
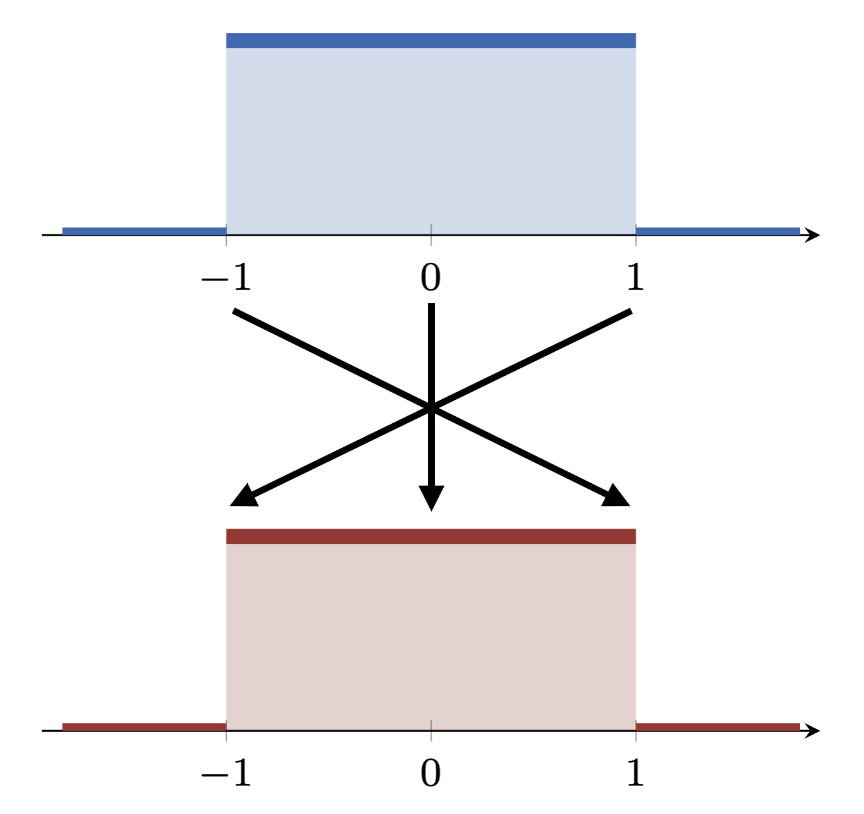
For the sake of this example, let us consider a population whose opinion distribution is uniform between -1 and 1 (blue distribution in the figure). Suppose now that we “flip the sign” of the opinion of each user: A user with opinion 1 now has opinion -1, a user with opinion -1 now has opinion 1, and so on.
Our goal is to understand this shift. At the level of individual agents, changes are indeed significant: We observe an average difference in opinions of 1, with extrema at 0 (for the users with opinion 0) and 2 (for the users with opinion +1 or -1).
However, nothing has changed at the population level: The opinion distribution is still uniform between -1 and 1 (red distribution in the figure). Thus, any reasonable notion of similarity will output a distance of 0 between the two distributions. This simple example illustrates that microscopic and macroscopic shifts do not always align. One direction always holds true: No microscopic change implies no macroscopic change. But, as this example shows, the converse might not hold true!
Of course, recommendation systems do not suddenly flip users’ opinions. Accordingly, our research investigates if and to what extent this discrepancy occurs when users interact in closed-loop with recommendation systems.
A Simple Model for the Closed Loop
Motivated by this simple example, our work investigates the possibility of such effects when the opinion of the users is affected by the interactions with a recommendation system.
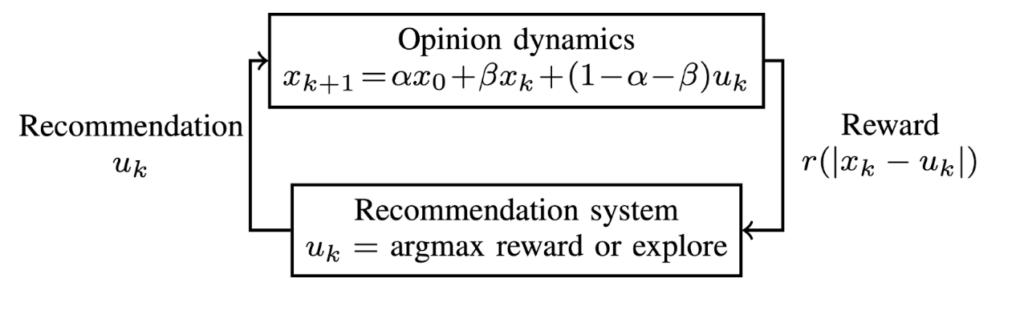
A model for users’ opinions
Our model of the users’ opinions can be explained with two simple parameters: A parameter, É‘, indicating the weight of constant user-specific prejudice has on the future opinion; a parameter, β, indicating the weight of the user’s current opinion on the future opinion. Accordingly, the weight of the recommendation received by the user on the future opinion is 1 – É‘ – β. If É‘ is close to 1, the user is very stubborn and unwilling to change their opinion; conversely, if É‘ and β are close to 0, the user is very susceptible to the content imputed by the recommendation system. This simple model, also known as the Friedkin-Johnsen model, is widely adopted to capture the time evolution of users’ opinions.
A model for recommendation systems
Instead, the recommendation simply outputs the recommendation that has yielded the highest reward (exploitation step), except for random recommendations at fixed intervals to look for better recommendations (exploration step). The length of the interval, T, which is the last parameter of our model, indicates how often the recommendation “explores” (i.e., selects a random recommendation from a given recommendation distribution to look for better ones).
Model Analysis
Our work investigates the effects of the problem parameters on the system’s evolution. We are mostly interested in two quantities: The average opinion shift across the population (measuring microscopic effects, i.e., how much has the opinion of an average user changed?) and the shift of the opinion distribution (measuring macroscopic effects, i.e., how much has the aggregate opinion distribution changed?).
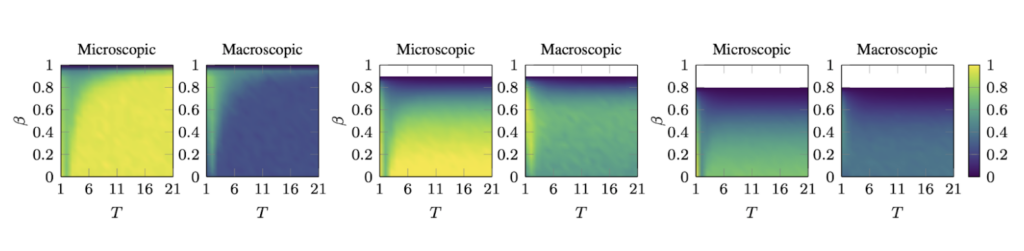
Notably, for small É‘ (two plots on the left, É‘=0), we observe a marked difference between microscopic and macroscopic effects: When one is the lowest (blue), the other one is the largest (yellow), and vice versa. As the weight of the prejudice increases (center, É‘=0.1), users are more stubborn, and this effect diminishes and eventually disappears (right, É‘=0.2). This suggests that, for moderately stubborn agents and long exploration times (i.e., for low levels of exploration-exploitation trade-offs), we observe what our initial example suggested: Little macroscopic shifts effectively mask considerable microscopic shifts.
Between the lines
Analyzing opinion dynamics has always held paramount importance, as it shapes individuals’ decision-making processes, influences political elections, and ultimately molds the socio-political landscape. Today, this undertaking becomes even more significant as the digital realm becomes inundated with automatically generated content distributed via recommendation algorithms that exert considerable influence over our consumption.
In this paper, we built a mathematical model of the closed loop between opinion dynamics and content recommendation. We examined the impact of exploration-exploitation parameters of recommendation algorithms on both aggregate distribution shifts and individual opinion drifts.
However, our study reveals gaps in the research, including the oversimplified representation of content recommendation algorithms and the need for a more nuanced model of human opinion dynamics. Moreover, the scarcity of empirical data limits our ability to validate these findings.
Addressing these gaps offers a promising avenue for future research, providing opportunities to integrate more realistic models of recommendation algorithms, refine our understanding of opinion dynamics, and collect empirical data. These future research directions will certainly deepen our comprehension of these systems’ role in shaping opinion landscapes and guide us through an increasingly dynamic information ecosystem.